
Hiking… trying not to fall off any cliffs that were washed out from last year.
From May 1 to now, I have hiked 393 kilometers (55 of those were mountain biking) to put up and take down remote cameras in Banff, Kootenay, and Yoho National Parks. I’ve hiked through hip-deep snow in early May, biked through overgrown trails with spruce branches smacking my shins, strolled along beautifully maintained and used trails along the river, hiker over mountain passes to remote valleys, and it’s all been amazing. This field work is really a gift in my life.
Anecdotal data
With every step I take in the woods, I’m thinking about how a bear is using this landscape and how to get the best data to capture that. Of course, we always put cameras where we think there is the best chance of catching bears and people in the same place, but sometimes it’s hard to tell. Sometimes it feels like a bit of a crap shoot – what if the bear walks behind the camera? Or around it? There are so many ways I might not capture the bear. But what if the bears aren’t there in the first place? What if they were in that valley last week but have since moved on?
The reality is I’m studying on the lowest density grizzly bear populations in North America. In the entire 20,000 km2 of my study area, there are less than 200 bears. Given this, it’s possible that I can just by chance set up a series of cameras on a trail when there aren’t even bears in the area at the time. I can’t really do too much about that. Where bears are depends on so many things that I can’t really predict, but it mostly depends on where and when the food is.
This past week, we set up remote cameras on a trail that is known to be high quality grizzly bear habitat this time of year due to an abundance of buffalo berries. But this year, there aren’t many berries on the trail. There were a few shrubs in berry in the higher elevations, but there weren’t any buffalo berries in the lower elevations. What was in the alpine wasn’t really enough to keep a grizzly bear happy for long. So now I wonder if I’ll really have any bears on these cameras that are in high quality grizzly bear habitat – not because of human use on the trails, but because for whatever reason there aren’t really any berries to eat in there this year.
This is andectodal information that isn’t data I’m formally collecting, but may end up being important when I sit down and try to make sense of things. It reminds me of all the confounding variables I have to consider… basically variables that confuse you and make you want to pull your hair out.
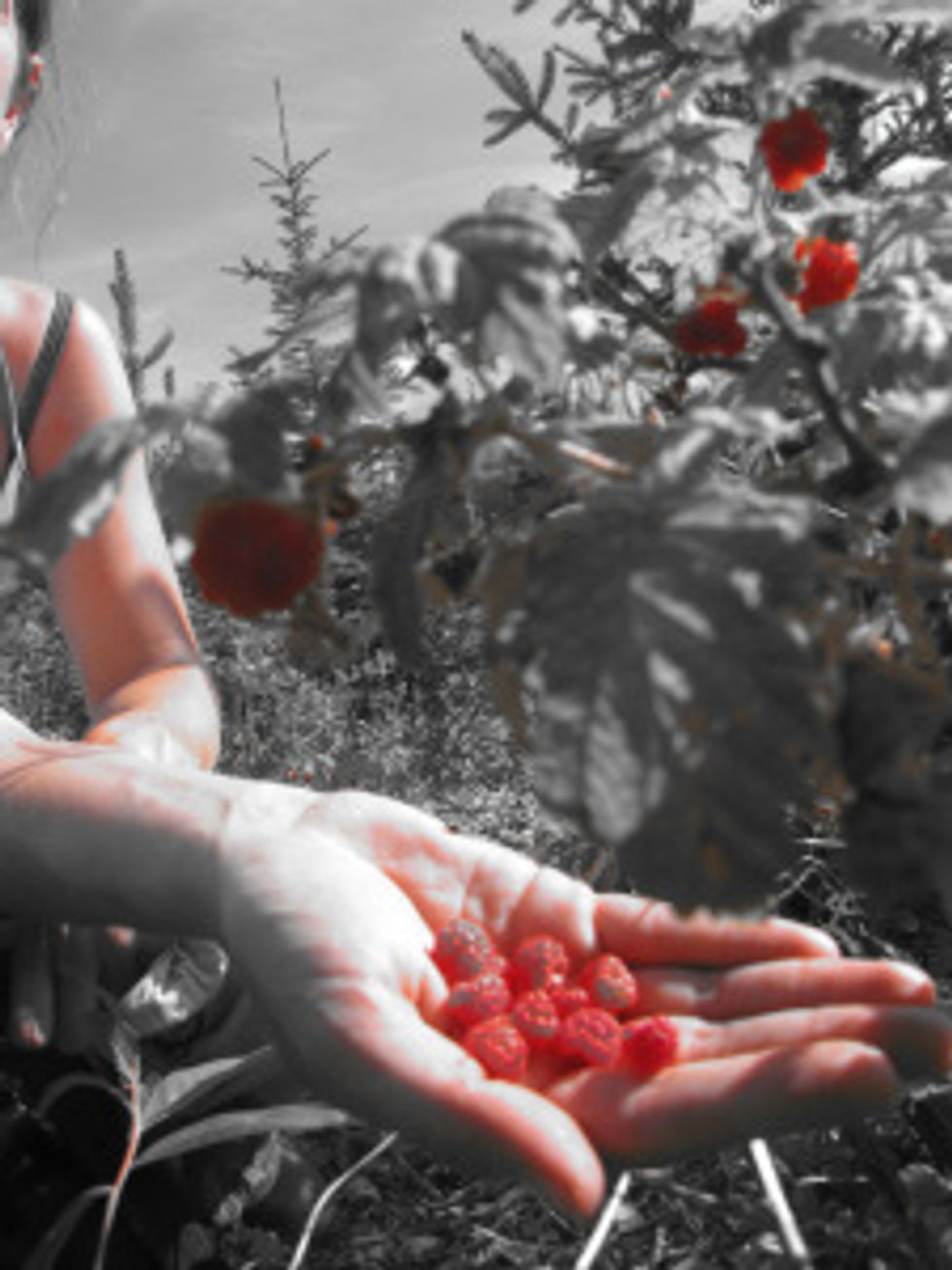
Abundant raspberries in an old clear-cut just outside of the Park boundary. What is a bear comes to snack here, but doesn’t walk past my camera in the Park less than 100m away?
Confounding variables
In statistics, a confounding variable is an extraneous variable in a statistical model that correlates with both the dependent variable and the independent variable (thanks Wikipedia). It’s basically something outside of your experiment that impacts the experiment but that you have no control over. In my case, I’m trying to examine grizzly bear habitat use in relation to human use on hiking trails. But just because there are or are not bears in an area doesn’t necessarily mean that’s because are or are not there. There are many reasons why a bear chooses to be somewhere: food, the presence/absence of other bears, the presence/absence of small cubs, food… did I mention food?
Bears are very food driven, especially at this time of year when they begin to enter hyperphagia (a period of face-stuffing to put on as much weight as possible before hibernation). We can pretty safely assume that a bear chooses where to be based on food first, and then weighs in other habitat attributes (including the presence of people) before deciding how long to spend in a place. Basically, food availability and human presence are on the opposite sides of a scale. Lots of food is where a bear wants to be, but as the number of people increases, the bear has to decide if the food is worth it. The tricky thing is that where that balance is struck is different for every bear. Each individual bear has a different level of tolerance for how many people can be in an area before the cost of accessing that food becomes too high and it’s better to eat elsewhere.
But that’s not all. If it was that easy, then my PhD would be simple: overlay the food availability (habitat layer) with human use and then just say where there’s lots of people it’s less likely that we’ll see this bear. I could actually do that for each individual bear and will be trying to do that later this fall (insert gasp of fear at trying to figure out how to do that in ArcGIS).
But now layer on top of that the fact that food resources change every year. What in one summer is a highly productive valley full of berries becomes a not so productive valley the next summer.
Now layer on top of that the impacts from the flood last year and see that what was once a highly used human trail isn’t so much this year because there are several wash-outs and no bridges; a trail that was easy travel has now become a technical hike.
Then layer on top of that various random events that haven’t even happened yet – extreme heat in one valley but not the next that impacts berry production, extreme thunderstorms or hail that smash a bunch of vegetation, late spring snow melt in the alpine and everything being a couple of weeks behind, a cold week of weather in August that halts berry production in some places. Many of these events are weather related. As a mountain woman, I know that snow can come at any time and I never go into the mountains without an extra layer of clothes and rain gear. As a researcher, I see that prolonged or intense weather events can have a dramatic effect on how and where berries are most abundant and available.
And all of these things impact where and when a bear is likely to be, potentially just as much or even more as the intensity and level of human use.

Each evening in the back country, I take some time alone to sit and think and stretch and maybe practice a little yoga. I mostly just need to reflect on what we’ve seen and what that might mean to the data.
What’s a biologist to do
Planning around and addressing confounding variables is not new to biologists. All biologists, whether in a lab or in the field, have to think about what the confounding variables are in their experiment and how to address them. It’s part of the methodological plan that you come up with before even entering the field or starting the experiment. To me, it’s the main reason why ensuring random sampling is so important. If the sampling design is random and there is a high enough sample size, I should be able to say that the confounding variables were essentially spread out over the landscape and that any differences I find are due to a relationship between the independent (human use) and dependent (bear use) variables. But that doesn’t mean that I can say there is a cause and effect relationship, just that they are related.
This is why I’m more than a little anal about the random sampling protocol and schedule, anything else could inadvertently introduce bias, which I would then have to figure out how to incorporate in to the analysis. As it is, I’ll have to be prepared to answer to these confounding variables in my analysis and discussion and defend the statistics I’ve used to flesh out any relationships I find.
The other thing I have to do is learn about things that lay on the periphery of the project scope. I have no idea why there aren’t berries in the lower elevations of some of these trails but there are a few berries in the higher elevations. Is it related to the few weeks of really hot weather we had? Or a lack of precipitation? Or how the berry bushes reproduce? Basically, I’ve got to do a little reading on buffalo berry and figure out what makes them tick. Just because I’m not seeing berries where I expected to doesn’t mean they aren’t out there in other valleys that I haven’t been hiking.
Learning, reading, thinking, learning, reading, thinking. That’s what a PhD is all about. Honestly, it’s one of the things I like most about it. It’s my job to read and learn, and then read some more. Pretty sweet.
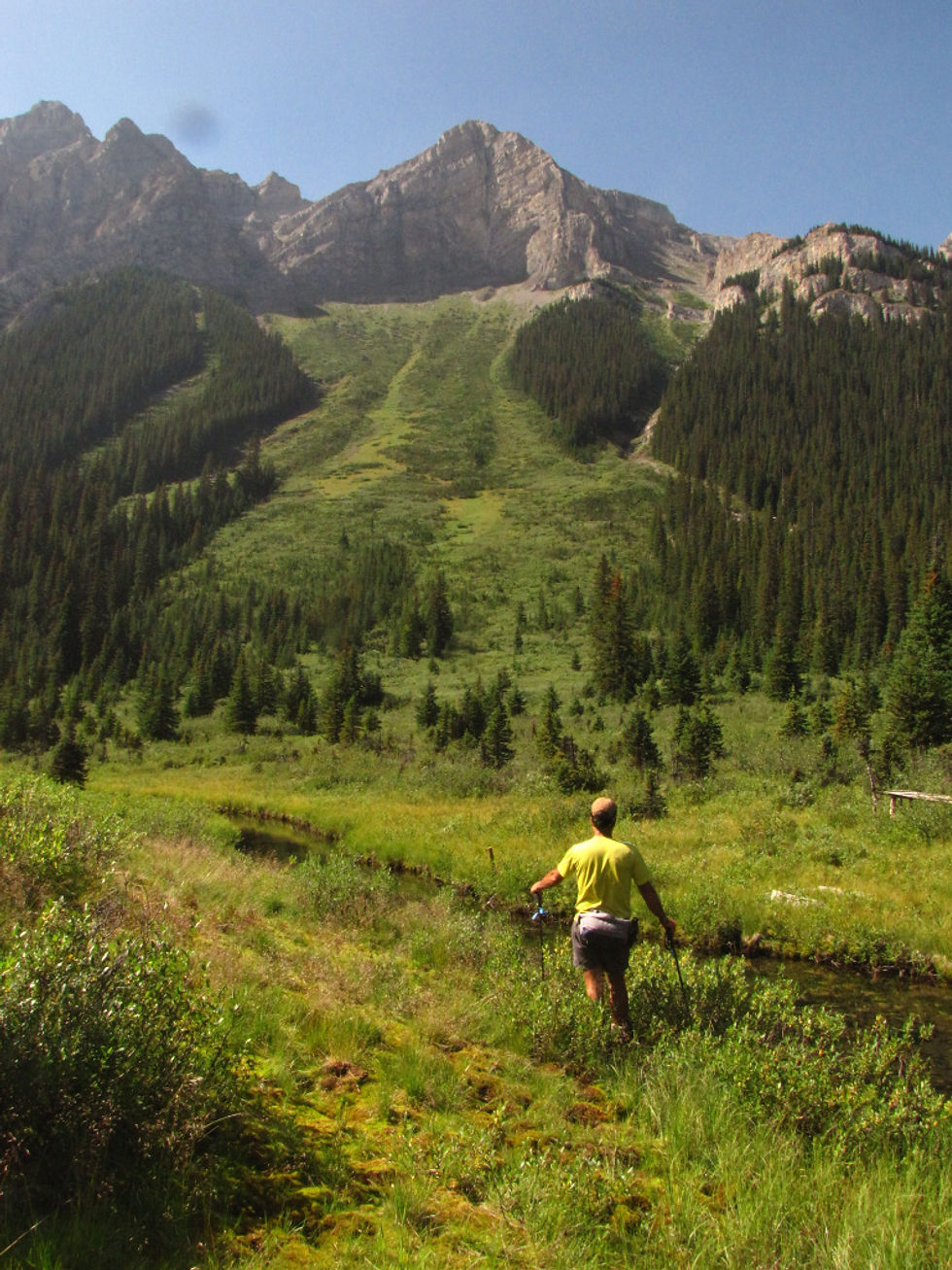
Basically, I walk and think, and walk and think… and then try to write it all down somewhere so I don’t forget.
コメント